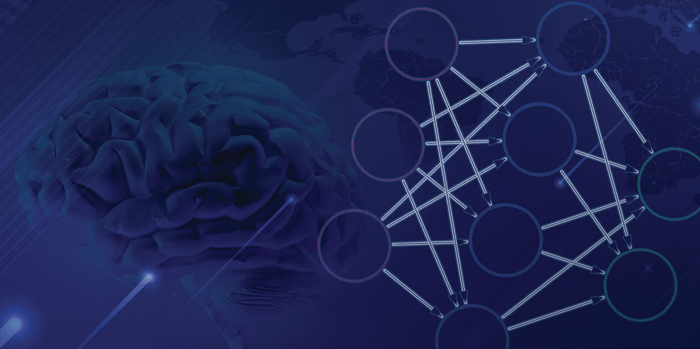
The fundamental idea of personalized learning is that each user has their own abilities, interests, and needs. The main goal of LightED Play is to build a complete profile (also called a model) of a user based on their ability levels (cognitive state) and to recommend resources (mini-games) according to their needs and abilities.
Recommendation methods have been used in the prediction of user performance. Researchers have shown that predicting user performance can be considered a rating prediction task since the user, task, and performance would become user, item, and rating in general recommender systems, respectively. (Nghe, 2012).
One of the most used methods in recommendation systems is Factorization Machines (Rendle, 2010), we use(d) it in LightED Play products due to its advantages are consistent with our products characteristics: a) it works well with very sparse data (not all users play all games), b) the amount of variables and datasets continually increases in time. (continuously new games and new user interaction are added to the products).
For example, Zoombers addresses the issue that mathematics can be considered a boring topic, because it often seems too abstract and not related to students' everyday life.
Zoombers' goal is to maximize students' engagement and enjoyment when they work with mathematical tasks. To pursue this goal, the Zoombers portal offers more than 300 games to reinforce (practice) mathematical skills. Intrinsic motivation due to game-based learning let the students interact not only with the set of games according to their own plan, but also with games recommended through intelligent algorithms that suit their current needs and abilities.
Recent analyses of game usage on the Zoombers portal show that:
- The greater the use of the games in the portal, the better the performance and the higher the skill metrics.
- The greater the use of the games recommended by the portal, the greater the measurement of related skills.